As the debate on generative AI and the need for guardrails heats up, we must look at the potential risks of these models of perpetuating old biases and barriers to gender equality.
In 2021 Google ethics researchers Emily M. Bender, Timnit Gebru, Angelina McMillan-Major, and Margaret Mitchell foresaw the risks of large language models (LLMs) in a paper titled ‘On the Dangers of Stochastic Parrots: Can Language Models Be Too Big?’
As a result, Google forced out both Gebru and Mitchell from leading the company’s Ethical AI team.
Two years later, Geoffrey Hinton has been able to normalize the discourse around the risks of AI in a way that was previously denied to female researchers.
More data means more biases
One of the risks of LLMs highlighted by the three Google ethics researchers is that the text mined to build GPT-3—which was initially released in June 2020—comes from sources that do not include the voices of women, older people, and marginalized groups, leading to inevitable biases that affect the decision-making of these systems. As stochastic parrots, these models are likely to absorb worldviews belonging to dominant groups from their training data. Harmful stereotypes against women and minorities risk being embedded in algorithms that are trained on datasets that do not represent all people.
As an example, mortgage approval algorithms have been found to be 40% to 80% more likely to deny borrowers of color than their white counterparts, according to an article reported by The Markup. The reason is the algorithms were trained using data on who had received mortgages in the past and, in the US, there is a long history of racial discrimination in lending.
How well do you really know your competitors?
Access the most comprehensive Company Profiles on the market, powered by GlobalData. Save hours of research. Gain competitive edge.
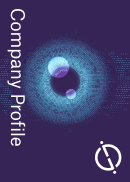
Thank you!
Your download email will arrive shortly
Not ready to buy yet? Download a free sample
We are confident about the unique quality of our Company Profiles. However, we want you to make the most beneficial decision for your business, so we offer a free sample that you can download by submitting the below form
By GlobalDataLLMs are inherently less accountable
Because the training data sets are so large, it is hard to audit them to check for these embedded biases. Indeed, the ethics researchers noted that “A methodology that relies on datasets too large to document is therefore inherently risky”. Language usage and nuances are especially important to promote social change, as the MeToo and Black Lives Matter movements demonstrate. The fact that AI models are trained on a huge amount of data scraped from the internet could fail to incorporate the anti-sexist and anti-racist vocabulary that movements have worked so hard to develop.
On a positive note, algorithmic accountability is being increasingly included in proposed regulations and frameworks. In the EU there is an ongoing debate around whether the original developers of general purpose AI models—those models that can have multiple intended and unintended purposes, such as ChatGPT—should be subject to the upcoming AI Act.
Despite some promising trends, female AI talent is still underrepresented
Women still lag behind men in digital and AI skills development. According to the OECD.ai Policy Observatory, in OECD countries, more than twice as many young men than women aged 16 to 24 can program, which is an essential skill for AI development. The good news is that female AI talent is growing faster than male AI talent, and the same research highlights that more and more women are joining AI R&D. But AI research is still dominated by men: in 2022, only one in four researchers publishing on AI worldwide was a woman. This not only limits women’s economic potential (as employers increasingly look to hire AI talents across different sectors), but it also results in women being less represented in AI publications worldwide and in the public debate around AI and its risks.
At a time when everyone is talking about AI risk management, it is critical to adequately address representation, bias, and discrimination issues of AI that risk undermining female empowerment.