Telefonica Tech’s recent announcement to partner with Sherpa.ai to offer federated learning addresses growing concerns about data privacy. The service provider announced that it will offer its customers Sherpa’s federated machine learning platform as well as professional services to help them deploy analytics and AI solutions.
With federated learning, models are trained locally, and only the results are transported and later aggregated and incorporated into a centralized model. The actual data is never shared, so compliance is less of a concern; and the data doesn’t leave its local environment, reducing data privacy concerns and the risk of data breaches. Telefonica Tech and Sherpa.ai will collaborate to develop industry-specific use cases, such as disease diagnosis for healthcare or fraud detection for financial services.
The move fits neatly into Telefonica Tech’s strategy to bring value added services to customers. The company already has several AI-related partnerships in place and offers multiple AI-based solutions. And conveniently, federated learning is well placed to drive demand for Telefonica’s higher bandwidth solutions, such as 5G private networking, since algorithm training requires large volumes of data and can be bandwidth intensive.
Telefonica Tech partnership is attractive
For sherpa.ai, the partnership brings a strong channel partner. Telefonica Tech is a major player in the IT services market in Spain, has a strong global presence, and provides access to a broad customer base. Furthermore, AI deployments can be complex undertakings and many organizations require more than simply a federated learning platform to get their projects off the ground. Telefonica Tech can provide customized consulting support via its professional services team.
Organizations continue to collect increasing volumes of data, some of it highly sensitive or subject to government regulations. Instead of moving this information to the cloud or to a centralized data center, enterprises are increasingly interested in exploring options for processing it near or at the point of generation or collection. Drivers of edge computing include the desire to maintain data privacy and reduce security-related risks, as well as to deploy latency sensitive applications or cut down on the cost of transporting data.
Federated learning benefits
The initial impetus for moving artificial intelligence (AI) processing to the edge was largely to support low-latency applications, such as computer vision for use on assembly lines or within AI-enabled cameras for security. However, organizations are now expressing interest in not only processing information at the edge using AI, but also in training machine learning models at the edge using federated learning. While federated learning offers numerous benefits, especially for organizations looking to leverage sensitive data, it is not without its challenges.
How well do you really know your competitors?
Access the most comprehensive Company Profiles on the market, powered by GlobalData. Save hours of research. Gain competitive edge.
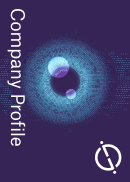
Thank you!
Your download email will arrive shortly
Not ready to buy yet? Download a free sample
We are confident about the unique quality of our Company Profiles. However, we want you to make the most beneficial decision for your business, so we offer a free sample that you can download by submitting the below form
By GlobalDataCompanies will need to sufficiently build out their edge infrastructure to ensure they can manage machine learning model training, and model transparency may be limited, since underlying data is hidden, making it more difficult to monitor models for fairness and identify unintended bias. As with all applications of AI, enterprises should carefully evaluate individual use cases, ideally with a multidisciplinary team, and ensure deployments align with corporate policies.
Related Company Profiles
Telefonica SA