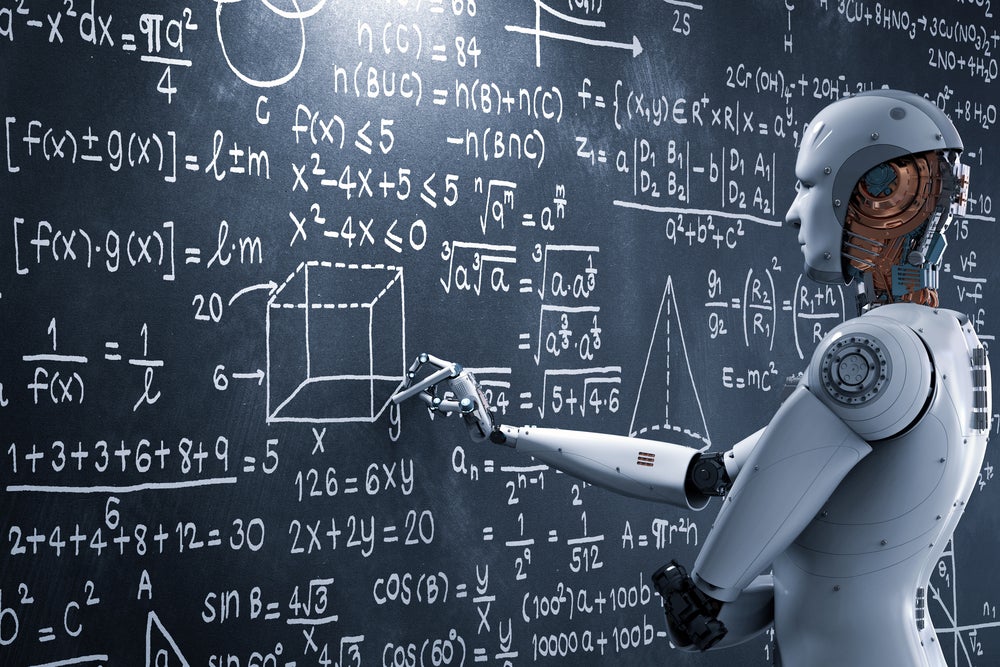
Machine learning is artificial intelligence (AI) technology which allows machines to learn by using algorithms to interpret data from connected ‘things’ to predict outcomes and learn from successes and failures. So what is machine learning in business and why is it important?
There are many other AI technologies, from image recognition to natural language processing, gesture control, context awareness and predictive APIs, but machine learning is where most of the investment community’s funding has flowed in recent years. It is also the technology most likely to allow machines to ultimately surpass the intelligence levels of humans.
For six decades, machine learning (ML) was poised to take off because members of the ‘artificial intelligentsia’ had already come up with the theoretical models that could make it work. The problem was that they were waiting for rich data sets and affordable ‘accelerated computing’ technology to ignite it.
These arrived in 2010.
Now, amid a swirl of hype, machine learning, software that becomes smarter as it trains itself on large amounts of data, is going mainstream, and within five years its deployment will be essential to the survival of companies of all shapes and sizes across all sectors.
Why does machine learning matter for business?
ML involves building computer algorithms which learn from existing data. Examples include predictive data models or software platforms that analyse behavioural data. Many start-ups have taken machine learning and applied it to specific industry verticals, such as detecting bank fraud or preventing a cyber-attack.
How well do you really know your competitors?
Access the most comprehensive Company Profiles on the market, powered by GlobalData. Save hours of research. Gain competitive edge.
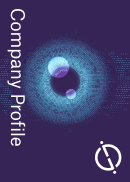
Thank you!
Your download email will arrive shortly
Not ready to buy yet? Download a free sample
We are confident about the unique quality of our Company Profiles. However, we want you to make the most beneficial decision for your business, so we offer a free sample that you can download by submitting the below form
By GlobalDataAI-infused solutions will become a high priority and a differentiator for businesses, driving demand for the skilled professionals that create them. However, the market faces a skills gap – there are few data scientists that specialize in AI relative to demand, and developing a model is a time-consuming and resource-intensive process. Solutions that automate and speed up model development and processing, while allowing data scientists to focus their limited resources on more specialized tasks, will help companies leverage the benefits of machine learning more quickly.
What are the big themes in machine learning?
High-end processors
Machine learning requires a lot of logic engines spread amongst a lot of high-speed, high-density flash memory. Within the last three or four years it had been found that the demands of neural net based deep learning could not be met at the processor level by high-end central processing units (CPUs). Hence the arrival from the gaming sector of graphics processor units (GPUs). Together, the combination of CPUs and GPUs can ‘accelerate’ deep learning and other forms of advanced analytics.
Cloud
AI is enjoying another boom after several false starts over the past three decades. Cloud computing has a fundamental role to play in democratizing AI by giving organizations access to the computing capacity required to run AI and machine learning algorithms.
Autonomous vehicles
Cloud, alongside in-vehicle data analytics and machine learning based on big data, is a fundamental technology in autonomous vehicles. Cloud computing has played an extremely important role in the development of autonomous vehicles, due to its ability to store and process the vast datasets generated by the numerous sensors, vision, pressure, temperature, and so on, associated with self-driving technology.
The leading car makers and technology companies are working to bring Level 4 fully autonomous vehicles, capable of handling entire journeys without human intervention, to market between 2019 and 2025. At Level 4 autonomy and beyond, the vehicle will have to carry its own data centre on board that can sense, infer, and act in real time, given the critical importance of zero latency in real-time road and traffic conditions. This onboard computer will also play a role in cordoning the vehicle off from external cyberattack. Edge computing will take over more and more of the work currently done in centralized cloud-based data centres.
Cybersecurity
In today’s digital economy, it is essential that companies of every stripe can collect, store, and adequately protect customer data and proprietary secrets. Traditionally, most companies have adopted a prevention-based approach to cybersecurity, but recent advances in technology areas like machine learning are enabling a move towards active detection of threats.
Organizations need to embrace cybersecurity as a core component of their digital transformation efforts and recognize the need to partner with third parties to establish the right cybersecurity strategy. Rather than reacting to threats as they arise, recent advances in technology areas like machine learning and behavioural analytics could give organizations the ability to detect potential issues at a much earlier stage, as well as freeing up resources that are currently occupied with analysing the constant flood of false positives generated by existing, more reactive systems.
Security software companies focusing on intelligence-led security solutions are likely to reap the biggest benefits in 2019.
Intelligence-led security
Enterprises desperately need reliable new sources of intelligence, not only to help accurately identify when an anomaly may actually be an attack but also to help with the constant flood of false positives. While still in its early stages, the power and potential of machine learning in support of behavioural analytics in enterprise security solutions is impossible to deny. Little wonder, then, that all the major enterprise security vendors are building, buying, or partnering to add machine learning in support of high-accuracy, intelligence-led solutions.
Machine learning has little value if a vendor doesn’t have a massive data set on which to apply the technology. This, in turn, will shift the enterprise cybersecurity market in a way that grants a significant competitive advantage to those vendors that not only build the largest dataset but also demonstrates the expertise to apply machine learning in such a way that it produces meaningful analytical insights.
Behavioural analytics
Drowning in a flood of security alerts, organizations struggle to discern real threats from harmless anomalous patterns. In too many cases, IT fails to detect an actual breach for days, weeks, or even longer. Behavioural analytics makes a case for using a number of different techniques, including machine learning, to comb through large volumes of data to identify attacks more accurately.
Online fraud
One of the biggest issues with online fraud is that it can go undetected for months, leading to sometimes serious financial losses and reputational damage. Online fraud prevention and detection software employ a variety of methods including identity authentication, machine learning, and behavioural analytics to identify threats and incidents early and mitigate damage.
As advances in areas like analytics and machine learning contribute to improvements in accuracy, online fraud detection software will become more effective. Unfortunately, cyber attackers will also employ more sophisticated tools, making it a never-ending race to outpace the enemy. More vendors will add professional services support, in areas such as forensic investigations, to further differentiate their offers and strengthen customer relationships.
Ambient commerce
Ambient commerce describes a new form of shopping which makes use of sensors coupled with AI to help customers select and pay for their goods without the need for keyboards or cash registers. For instance, Amazon’s Go stores, of which there are currently four, offer a vision of frictionless commerce, where computer vision, sensors, and machine learning technologies enable customers to ‘grab and go’. By contrast, Alibaba’s Hema stores offer a vision called ‘New Retail’ in which customers use smartphone apps combined with QR codes to shop and go.
Shopping remains a predominantly offline affair. The companies that will flourish will be those that have at their disposal the keenest, most up-to-date datasets about human shopping behaviour in general and their own existing and target customers in particular. These companies will typically embed ambient computing and vast arrays of sensors in their stores linked to analytical and machine learning algorithms. Ambient commerce will also drive expenditure on IoT connected devices and on IoT software and services in the retail sector.
Advertising
The main challenge that advertisers will face in 2019 is tighter regulation of the digital advertising market. Data privacy, data protection, fake news, copyright, and tax avoidance are all areas that regulators are likely to target over the next 12 months. At the heart of the regulatory debate is the question of whether the big tech giants, like Google, Facebook, and others, should retain their legal status as neutral content aggregation platforms, which are not subject to heavy regulation, or be re-classified as publishers, which are.
Effective tax rates for some of the larger tech giants in the digital advertising space may also rise as governments all over the world mull over the concept of taxing local revenues rather than local profits.
While big tech companies are better equipped and more diversified to navigate these uncertain times, their compliance costs are almost certain to rise. For example, they may be required to build more local data centres.
This year Google unveiled some AI-based tools to help advertisers develop more effective ad campaigns. They range from responsive search ads that use machine learning to mix and match content, to optimizing ad performance on YouTube by automatically adjusting bids.
Ad fraud
The smartest advertisers are adopting AI-based solutions to counter ad fraud. Many use machine learning algorithms to detect fraud patterns, based on suspicious patterns of behaviour.
Unsavoury content
Unsavoury content, such as terrorist propaganda, pornography, or racist material, poses a serious challenge to social networks and web search companies. In 2018 YouTube was forced to increase moderators to 10,000 to combat unsavoury content. Facebook now has 7,500 moderators, up from 4,500 in 2017. Using AI technologies, including machine learning, image recognition, video recognition, context awareness and speech and text recognition, Google has gone some way towards solving this problem. Facebook, whose AI is not as good as Google’s, is taking a more manual and therefore costlier approach. As a result, some of the furore around this subject has died down and many of the big global brands have come back to Google.
Voice
Voice will soon become one of the default interfaces used to interact with applications, alongside keyboards, mice, and touch screens. Voice technology requires chips optimized for machine learning rather than traditional processors, such as Intel microprocessors, which are not optimized for voice analysis.
Edge computing
An example of edge computing is Apple’s Core ML software. Typically, machine learning takes place in a large data centre, but core ML allows machine learning to take place on the iPhone itself instead.
What is the history of machine learning?
AI languished for six decades, kick-started by a series of famous conferences in the 1950s. Despite the brilliance of the participants, the AI industry overpromised on the scope and prospects for executing a series of leapfrogs to achieve the end states envisaged by leading mathematicians such as Turing and Von Neumann.
In the event, founder members of the artificial intelligentsia, such as John McCarthy and Marvin Minsky were hamstrung by a lack of affordable advanced computing power and rich real-time data sets of structured and unstructured data on which to run their algorithms.
This changed in around 2010 by when compounding advances in accelerated computing and the emergence of huge real-time data sets from social networks, search, media and sensors enabled an AI marriage made in heaven.
Until then progress in AI/ML had been slow. It progressed from working in first-order logic to the development of expert systems and then mature statistical, increasingly Bayesian, analysis.
The big breakthrough came in the development of deep learning algorithms based on artificial neural networks, or ‘neural nets’, suitably hosed by rivers of data, as famously exemplified by Google’s AlphaGo programme which works out almost every permutation and a combination of sequences in the Chinese board game of Go.
This is yielding major advances in computer vision, image and object recognition and semantics, and natural language processing.
Progress is now exponential.
These deep learning algorithms hold out the clear promise of truly smart, increasingly pre-cognitive and companionable ‘conversational’ virtual agents, smart sentient robots and autonomous vehicles.
And it’s not just the tech titans developing and deploying these neural nets. Automotive sub-system component suppliers are doing so as a means to control their semi-autonomous driving platforms.
More and more products, devices and services will understand and obey voice or gesture commands, and eventually brain frequency commands.
The world of artefacts will have eyes, ears and haptics, touch sensors.
The story of machine learning technology …
- 1642: Pascal invents the first digital calculator
- 1859: Babbage and Lovelace work on steam driven programmable calculating machine.
- 1913: Whitehead and Russell revolutionise formal logic in Principia Mathematica.
- 1948: Von Neumann proves that a general computer can simulate any effective procedure.
- 1950: Alan Turing develops the Turing Test to assess a machine’s ability to exhibit intelligent (human-like) behaviour.
- 1952: Arthur Samuel coins the term ‘machine learning’ at IBM and writes first game playing program for Draughts (Checkers).
- 1956: Phrase ‘Artificial Intelligence’ first aired at McCarthy’s Dartmouth Conference.
- 1959: McCarthy and Minsky form the MIT AI Lab.
- 1965: Weizenbaum (MIT) builds Eliza interactive program based on natural language dialogue (English)
- 1973: Lighthill Report heavily critical of AI research sets matters back in UK and US.
- 1997: IBM’s Deep Blue defeats world chess champion, Kasparov.
- 1998: Berners-Lee publishes landmark Semantic Web Road Map paper.
- 2005: Tivo invents recommendation technology based on tracking web activity and media usage.
- 2009: Google builds first autonomous car.
- 2010: Microsoft Kinect for the Xbox first gaming device tracking human body movement.
- 2011: IBM Watson beats human champions in TV game show, Jeopardy, demonstrating AI that understands sophisticated nuances.
- 2011: Natural language based virtual assistants appear—Siri, Now, Cortana.
- 2014: Tesla introduces AutoPilot, software which is later upgraded for fully autonomous driving.
- 2014: Amazon launches Echo, its intelligent voice-activated speaker, powered by Alexa, its AI engine.
- 2015: Baidu launches Duer, its intelligent assistant
- 2016: Google DeepMind AlphaGo algorithm beats world Go champion Lee Sedol 4-1.
- 2017: Libratus, designed by Carnegie Mellon researchers, beats top 4 players in ‘no limit Texas hold ‘em’ poker.
- 2020: AI becomes the new ‘electricity’ – developers plug into machine learning APIs for a wide variety of apps.
- 2025: Lethal cyber-attacks on connected cars, infrastructure and medical devices.
- 2030: The general application of AI/ML gradually turns the world into a computer-generated Matrix.
- 2045: 50% probability of full human-level AI, according to a poll of AI experts, source: Muller & Bostrom 2014.
This article was produced in association with GlobalData Thematic research. More details here about how to access in-depth reports and detailed thematic scorecard rankings.