On October 1, 2024, MOSTLY AI announced that its platform can help enterprises create synthetic text, a timely new capability given the growing interest by enterprises to leverage GenAI to extract insights from unstructured data.
With MOSTLY AI’s new capability, customers use a combination of proprietary models from MOSTLY AI and open-source GenAI models from HuggingFace to fine tune an LLM and create statistically accurate synthetic text. The quality of the output data is enhanced by the use of structured data. The resulting synthetic text can then be used to customise GenAI-driven applications.
What is synthetic data?
Synthetic data is information created by generative AI technology that is statistically similar to actual data. It is an attractive and increasingly popular option for organisations that need more data than they have readily available to train machine learning models or that don’t want to use actual data to train models because of privacy concerns.
Synthetic tabular data is already being used to train models, test software quality, and support staging and demo environments. Similarly, synthetic unstructured data, or text, can be used to train and fine tune LLMs used in customer support applications or chatbot conversations. And while there is always the option of manually creating data, the process is time-consuming and resource-intensive, making synthetic data an appealing alternative.
The use of GenAI
According to Rena Bhattacharyya, Chief Analyst and Practice Lead for Enterprise Technology and Services at GlobalData, “Over the past several years, much of the conversation around synthetic data has focused on using GenAI to create synthetic tabular data. Tabular data is structured data that can be neatly organised, for example information that can be arranged in an Excel file. The logical next step is to use GenAI to create text-based information that can be used to customise large language models (LLMs).”
Synthetic text and MOSTLY AI
MOSTLY AI is already well positioned to help organisations with their synthetic unstructured data needs. The Vienna, Austria-based company was founded in 2017 and is a well-known player in the synthetic data market. It has received $31m (€28.5m) in funding from European venture capitalists.
How well do you really know your competitors?
Access the most comprehensive Company Profiles on the market, powered by GlobalData. Save hours of research. Gain competitive edge.
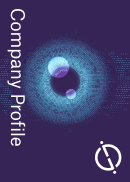
Thank you!
Your download email will arrive shortly
Not ready to buy yet? Download a free sample
We are confident about the unique quality of our Company Profiles. However, we want you to make the most beneficial decision for your business, so we offer a free sample that you can download by submitting the below form
By GlobalDataMOSTLY AI designed its platform with ease of use in mind, making it accessible to those that aren’t data scientists or data engineers. For those that want to experiment with the technology and aren’t ready to commit to an enterprise license, which includes SLAs related to customer support, the company also offers a free tier of services.
Challenges
There are, of course, challenges when it comes to working with synthetic data, the most notable of which is quality concerns. Various techniques and platforms result in data that can range in accuracy. Organisations will need to evaluate their synthetic data and take advantage of quality assurance reports.
One best practice is to train one model using actual data, train another with synthetic data, and test the resulting models with actual data withheld from training, and to compare results. Furthermore, even synthetic data may not be fully anonymous, a challenge users should be aware of. To tackle this problem, organisations should seek out platforms that offer tools that evaluate results, including outliers.
The future of synthetic text and data
Going forward, the application of synthetic data, both tabular and unstructured, will continue to grow, driven by a need for additional training data as well as concerns over data privacy.
Though some organisations remain wary of using synthetic data, new tools are chipping away at remaining obstacles, making the solution a more attractive and attainable option. Evolving regulatory requirements will drive further momentum. However, there is still much need for education in this area since most organisations are only just getting started with the adoption of synthetic data.