Artificial intelligence (AI) is rapidly evolving, and the rise of small language models (SLMs) marks a shift in the landscape.
SLMs, typically defined as models with fewer than 10 billion parameters, offer several advantages compared to large language models (LLMs), in terms of cost, efficiency, and adaptability.
What are SLMs?
SLMs are designed to be easier to train, deploy, and adopt than LLMs. By using smaller and more focused datasets, training times for SLMs are reduced, often taking just weeks compared to the months required for LLMs. This allows organisations to deploy AI solutions tailored to specific needs and cost-effectively.
The compact nature of these language models makes them well-suited to real-time, and environments with limited compute resources such as mobile applications. With fewer parameters, these models have shorter inference times, thus reducing latency.
Moreover, SLMs require significantly less computational power, resulting in lower operational costs and reduced carbon footprints. This “greener” approach to AI makes SLMs attractive to companies prioritising sustainability and cost-efficiency.
Security and regulatory benefits
SLMs offer enhanced security compared to LLMs. Their smaller datasets reduce the attack surface for cyber threats, simplifying data protection and enabling faster vulnerability identification. Additionally, SLMs can be operated locally, minimising the risks of data breaches and improving data privacy.
How well do you really know your competitors?
Access the most comprehensive Company Profiles on the market, powered by GlobalData. Save hours of research. Gain competitive edge.
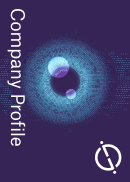
Thank you!
Your download email will arrive shortly
Not ready to buy yet? Download a free sample
We are confident about the unique quality of our Company Profiles. However, we want you to make the most beneficial decision for your business, so we offer a free sample that you can download by submitting the below form
By GlobalDataThe regulatory landscape around AI models is growing more complex, with increasing scrutiny on large-scale data handling and energy usage. SLMs, due to their smaller size, avoid many of the stringent obligations that apply to LLMs. For instance, they don’t meet the high computing thresholds often tied to stricter regulations, reducing compliance costs for enterprises.
The market impact of SLMs will be high
While SLMs are not a replacement for LLMs, they are a powerful complement, offering tailored solutions for specific industries and applications. As the AI market grows more competitive, organisations are under pressure to demonstrate clear business value and ROI.
The reduced training costs, increased energy efficiency, and many regulatory advantages of these language models are set to drive their adoption across industries. SLMs, with their suitability for industry-specific applications, offer easier scalability across diverse environments.
Numerous companies are developing SLMs. There is increasing pressure for competitors in the generative AI market to demonstrate tangible use cases for applications of the technology. GenAI providers such as Microsoft, Meta, and Google have all recently released SLMs, but also Hugging Face and Mistral in collaboration with NVIDIA. Interestingly, we are starting to see the first real-world applications.
For example, India has launched LexLegis.AI, its first legal-specific AI platform. Developed in Mumbai, it trained a model on over 10 million Indian legal documents, including court judgments and statutes. LexLegis.AI aims to make legal research faster and more precise, tailored specifically for India’s complex legal system.
SLMs act as a bridge between complex, resource-heavy AI and enterprises offering scalable solutions. By offering tailored capabilities with lower costs and faster deployment, they make advanced AI accessible for diverse business needs.
Related Company Profiles
Microsoft Corp
Meta Platforms Inc
Google LLC
NVIDIA Corp