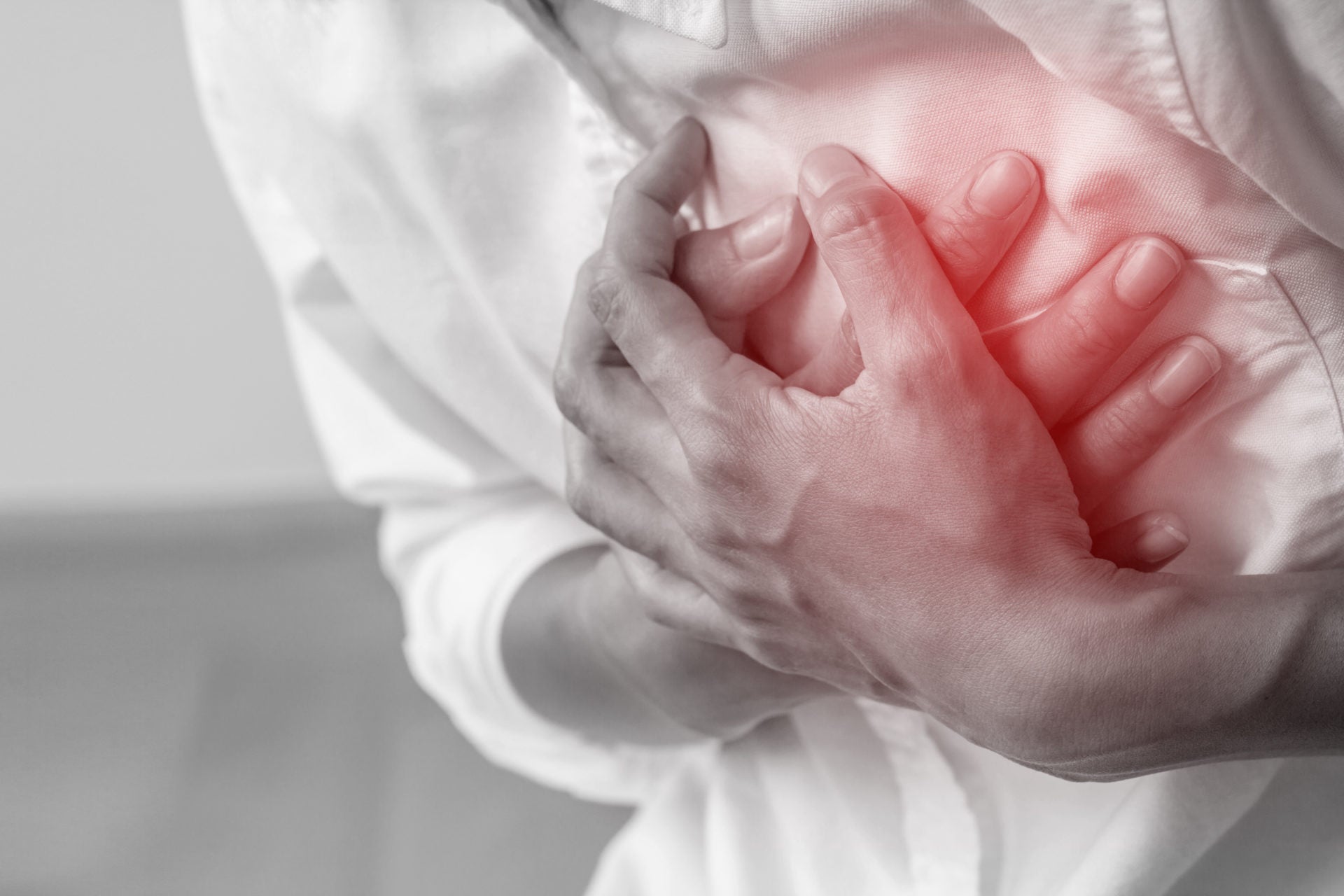
A machine learning algorithm has predicted heart attacks and death from heart disease with 90% accuracy, overtaking human practitioners.
Using data from 950 patients with chest pain, researchers from the University of Turku, Finland, were able to train an algorithm to identify patterns that indicate a higher propensity for heart attacks and cardiac-related deaths.
The outcome of these patients was known over a six-year period, which meant the researchers were able to know with certainty if the predictions were accurate.
Being able to predict the likelihood of a patient suffering a heart attack could help medical practitioners better personalise treatments. About 610,000 people die of heart disease every year in the United States, according to the Centers for Disease Control and Prevention.
Study author Dr Luis Eduardo Juarez-Orozco, of the Turku PET Centre, Finland, said: “These advances are far beyond what has been done in medicine, where we need to be cautious about how we evaluate risk and outcomes. We have the data but we are not using it to its full potential yet.”
The findings were presented yesterday at the International Conference on Nuclear Cardiology and Cardiac CT (ICNC) in Lisbon, Portugal.
How well do you really know your competitors?
Access the most comprehensive Company Profiles on the market, powered by GlobalData. Save hours of research. Gain competitive edge.
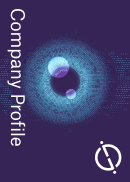
Thank you!
Your download email will arrive shortly
Not ready to buy yet? Download a free sample
We are confident about the unique quality of our Company Profiles. However, we want you to make the most beneficial decision for your business, so we offer a free sample that you can download by submitting the below form
By GlobalDataHow machine learning predicts heart attacks
To test the algorithm, researchers began by gathering patient data that could indicate coronary artery disease. Using a coronary computed tomography angiography (CCTA) scan, researchers gathered 58 pieces of data on the presence of coronary plaque, vessel narrowing and calcification.
Patients who appeared more likely to have heart disease went through further testing where a further 17 data variables were gathered.
There were 24 heart attacks and 49 deaths during an average six-year follow-up of these patients.
Knowing the outcome, researchers were able to enter all 85 variables into the machine learning algorithm.
The algorithm repeatedly analysed these variables, looking for patterns in the scans that indicated a higher propensity for a heart attack.
Because the outcome is already known, researchers can clearly measure the algorithm’s accuracy.
Dr Juarez-Orozco said: “The algorithm progressively learns from the data and after numerous rounds of analyses, it figures out the high dimensional patterns that should be used to efficiently identify patients who have the event. The result is a score of individual risk.”
She added that humans find it difficult to think in the many dimensions needed to predict outcomes in individuals.
“Doctors already collect a lot of information about patients – for example those with chest pain,” continued Dr Juarez-Orozco. “We found that machine learning can integrate these data and accurately predict individual risk. This should allow us to personalise treatment and ultimately lead to better outcomes for patients.”
The ICNC is co-organised by the American Society of Nuclear Cardiology (ASNC), the European Association of Cardiovascular Imaging (EACVI) of the European Society of Cardiology (ESC), and the European Association of Nuclear Medicine (EANM).
Read more: Continuous health monitoring is the future of healthcare, study finds