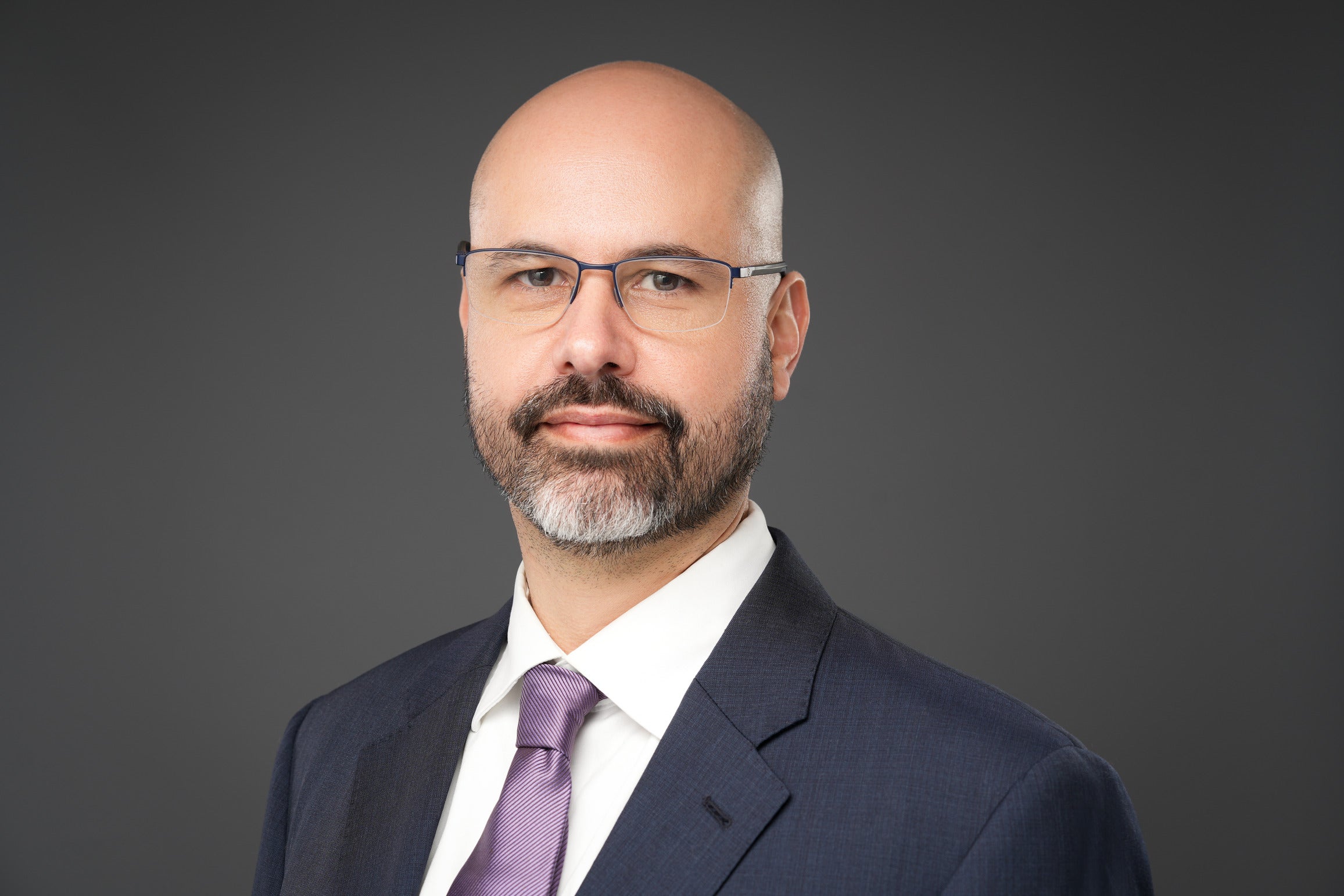
In broad terms, what is the integration of AI into radio area networks (RAN)?
There are two angles to the integration of AI and RAN. One is how can we use AI to have a better radio access network? In fact, everywhere I look at Mobile World Congress (MWC) I see examples of this. New capabilities being deployed include channel estimation, for example, something that the network needs to do to know which radio channel to use to talk to a user’s device. This is something that happens very fast, in an automated fashion already, but with AI in can be done much quicker with users accessing a higher quality channel.
The second angle is the flip side of the same coin and is what the radio access network can do for AI workloads so that users can have a better experience and better service. These are both AI and RAN integration.
But there is also a third aspect that’s a little more technical. As we evolve the types of computers that we use to run the RAN, they become more similar to the types of computers on which we develop AI. Eventually that means we can share the same infrastructure with a greater ability to allocate capacity. This depends on what volume of the network is being used the most at any point in time.
Typically, RAN networks are designed and dimensioned by the peak hour. In other words the size of the network, or the capacity of the network, determined by the time of the day or the time of the week which most people are using it. It also means that a lot of time, there is spare capacity. By integrating AI into the radio
access network, that spare capacity starts to be able to be used to serve AI workloads as well.
We can view these three aspects as: AI for ran (AI working for the radio access network), AI on RAN (running on the radio access network), and AI and ran (when the two capabilities share the same infrastructure).
What is your advice to mobile operators in terms of opportunities that AI RAN might present?
Network operators have to be obsessed about the total cost of ownership. I think that’s going to be a large component of the benefits they capture from AI. But not all the benefit. Opportunities will come from providing different and better services to users and monetising that accordingly.
Can AI-RAN change the telecoms business model with new revenue streams?
It’s very possible and we are anticipating this. The reason relates to the third category of AI RAN that I mentioned, using the same infrastructure for RAN and for AI workloads. In fact, for a significant percentage of the time the radio access network is underutilised. If we find ways to essentially serve third-party AI workloads, then this is arguably a new revenue stream.
What challenges do mobile operators have in adopting AI RAN?
One challenge that mobile networks have is the renewal cycle. There is a natural renewal of equipment because people innovate, new equipment becomes available, and you want to upgrade. And then there is also the changing of generations. Changing generations, historically, happens every ten years or so. Some of the more transformational changes in the telecoms business model come with these cycles.
And because of that, sometimes it’s very difficult for mobile operators to anticipate demand during generational change, and they end up with a lot of spare capacity. This means investment comes ahead of the demand. With AI RAN and AI network virtualisation and OPEN RAN, as well as some other recent developments, it becomes easier to make improvements in a more staggered manner.
And in this way, operators can track new customer demands more closely and more easily, so they don’t have to invest too much ahead of the coming demand. It’s good for any business model if you can have the investment cycle and the monetisation cycle more closely aligned
Are there different challenges in different regions?
Network topologies can be very different as cities are organised differently. So, it’s hard to compare. Because of that, the challenges are unique depending on the way the networks are built in the first place. So, frankly, I don’t think a comparison makes much sense. What you’re trying to do in each case is to figure out the best possible way to serve both the network and the AI workloads across all the different use cases.
If we have more specifically tuned building blocks, then each operator can combine them in the way that makes more sense for their customer base. That’s another way to say that this will require a lot of collaboration and partnership.
Does anything stand out at MWC for you this year?
I saw a lot of AI-RAN, but partially because that’s my focus. There are quite a few different approaches and different players who are doing things in this space. This doesn’t surprise me, because of the traction that we’re seeing on AI. If you look at the supply side, it offers capabilities that weren’t there for the network before.
On the demand side, users want to do new things many involving AI, and we’re looking at ways to serve that demand. So, trying to connect users with the best possible technology for them at any point in time, and MWC is usually the time of year where that happens more with more intensity.