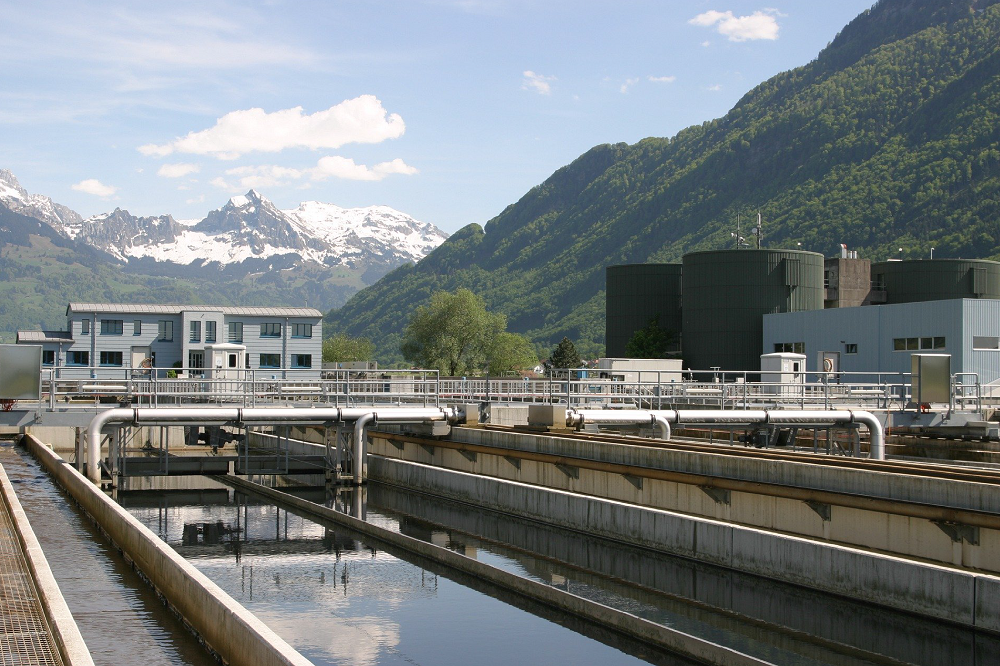
The increasing integration of artificial intelligence (AI) to support company operations is trending across all kinds of sectors, from government agencies to the medical field. Vice president of AI at Innovyze, Thouheed Gaffoor, observes that key market indicators, including acquisitions and early-stage investments, are showing high levels of activity in the AI space by big data companies and beyond, despite the pandemic. Meanwhile, statistics from PEGA show that 67% of companies are already using AI to support decision-making.
The water industry is no exception. There is a “strong value proposition” for AI deployment in this sector, affirms James Legue, director of AI products at Innovyze.
Value proposition
“There are two real domains where AI helps water,” explains Gaffoor. “One being operations and the other asset management.” The integration of AI into operations can optimise both predictive control and preventative management. “Predictive control is all about how we can operate our infrastructure or processes more intelligently,” Gaffoor adds. “Preventative management is more about how to anticipate potential issues and respond to them as proactively as possible.”
Legue emphasises that facilities operators make many operational decisions on an hourly basis. The quality of these decisions is subject to human factors, and it becomes more difficult when the decision-maker is faced with challenges like extreme weather events or outdated infrastructure. Integrated AI takes the pressure off the individual making decisions. An AI system can receive vast amounts of data from relevant sources – like weather forecasts or energy tariffs, for example – and makes decisions based on all the data it processes.
Balancing savings with compliance
“Emagin is an AI platform designed to help water, waste-water and industrial facilities make better operational decisions in real time,” says Gaffoor. Real-time data within the system and its environment flow into the model, which makes recommendations on how the system can be optimised.
Two key objectives of the Emagin operating model are to help customers make savings in terms of their energy and chemical consumption, while maintaining compliance. For the manager of a wastewater treatment plant, increasingly strict regulation, combined with evolving environmental conditions, makes it difficult to optimise operations while maintaining compliance. For example, out of fear of being fined by the regulator, the plant may overuse chemicals and energy to maintain effluent quality. “They may not have the right data to understand the right chemical dosage to meet compliance,” explains Legue. Emagin can recommend the optimal chemical dosage based on an incoming load and external and system conditions, while controlling effluent quality for compliance. This has huge value for operations including water treatment, mining, paper and pulp and beverage production.
How well do you really know your competitors?
Access the most comprehensive Company Profiles on the market, powered by GlobalData. Save hours of research. Gain competitive edge.
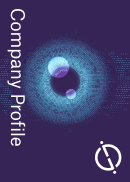
Thank you!
Your download email will arrive shortly
Not ready to buy yet? Download a free sample
We are confident about the unique quality of our Company Profiles. However, we want you to make the most beneficial decision for your business, so we offer a free sample that you can download by submitting the below form
By GlobalDataThe value translates directly into savings. Through energy savings, chemical savings and downtime reductions, “we can guarantee six-month payback year over year,” says Legue.
For water companies hoping to deploy an AI system, “it all starts with understanding your level of readiness,” says Gaffoor. To bring AI into a plant, Innovyze looks at the existing instruments and data infrastructure. It later walks customers through the installation process, and “ensures that the AI project goes into production and starts generating real value through cost savings,” Gaffoor explains.
Two future trends
Looking ahead, Gaffoor identifies two major trends in AI deployment. The first concerns machine learning (ML) operations. “Many AI companies have been positioning themselves to provide more production-ready ML technologies, making sure you have a life cycle that can build trade models faster and get them to production,” he explains. This avoids AI solutions getting stuck at the R&D stage.
Secondly, there is increasing demand for explainable AI (XAI), where there is clear traceability of the system’s decision-making process, from input to output. “If you take both of these concepts and think about how they work together in industrial settings, that’s a really powerful tool to bring to the market,” adds Gaffoor.