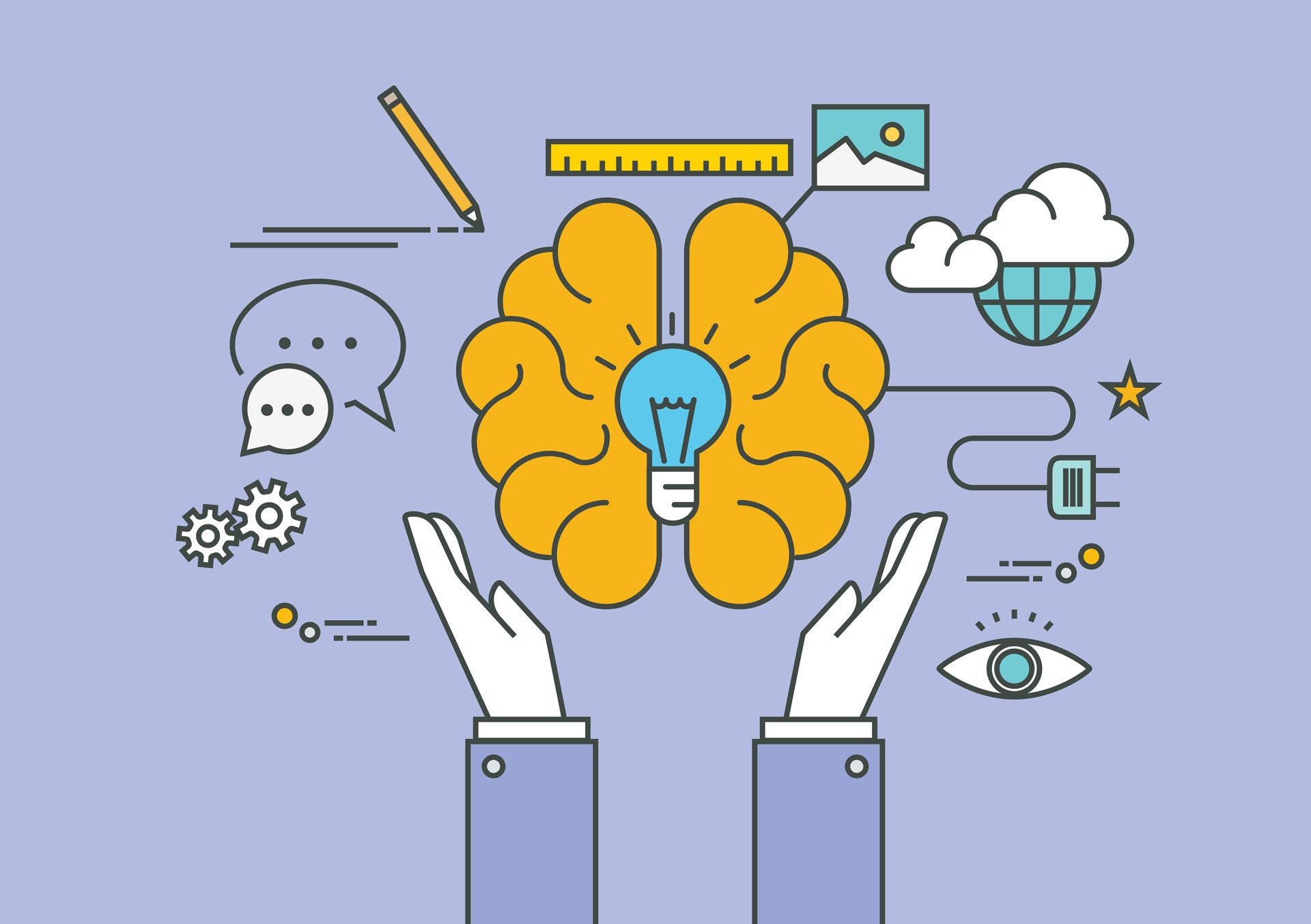
Every great idea starts with a question. What if we were to…? How can we…? Why don’t we…?
Sometimes, the problem (especially if you’re not a formally trained data scientist) is knowing what question to ask. And more importantly how to ask it. That’s where design thinking comes in.
A good question usually cascades into more questions that result in a better answer and a better outcome. But how does and how should our thinking change as automation becomes more prevalent and machines start to make more decisions? Let’s start from square one…
Design thinking for data science
All great innovators in literature, art, music, science, engineering, and business have practiced design thinking. Chances are, you’ve used it at some stage in your career without even knowing it. It’s a method in ideation and development that describes a human-centred, iterative design process consisting of five steps: empathise, define, ideate, prototype and test.
It’s often compared to, and aligned with, agile development practices. Design thinking has been used for years to tackle problems that are ill-defined or unknown, which makes it the perfect methodology for data scientists and business analysts tackling big business problems with little direction and an abundance of data.
Design thinking at its core has remained the same in principal and objective, but it has been forced to evolve over the years as new developments in business strategy and technology have emerged to influence our thinking and decisioning.
How well do you really know your competitors?
Access the most comprehensive Company Profiles on the market, powered by GlobalData. Save hours of research. Gain competitive edge.
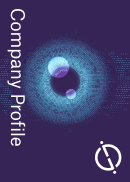
Thank you!
Your download email will arrive shortly
Not ready to buy yet? Download a free sample
We are confident about the unique quality of our Company Profiles. However, we want you to make the most beneficial decision for your business, so we offer a free sample that you can download by submitting the below form
By GlobalDataFor those of us working with data, the continued advancements of machine learning and artificial intelligence technology will undoubtedly impact how we uncover insights for our organisations, but one thing is for certain: Humans will always be a lynchpin in design thinking, and design thinking will always be a lynchpin in finding the best answers.
Getting to (wh)Y: Design thinking to uncover what machines cannot
If you read the headlines today, everyone is afraid that machines are going to take their jobs.
It’s true, automation and technology are changing the modern-day workforce, but humans are still, and will continue to be, a critical part of all technology and automation. Technology and automation are simply enhancing human’s ability to make a decision and move faster, but they’ll never replace the need for human intuition and experience – two critical facets of design thinking.
I recently detailed a real-life experience that illustrates this point perfectly and highlights why it is so important for human involvement, particularly when it comes to data science teams and problem solving.
At a high-level, a team of data scientists was engaged by a manufacturing organisation to optimise staffing to keep an assembly line moving. The team came together and developed a pristine predictive analytical model that suggested that the company could lower number of staff members on the line through more precise scheduling. It failed… Why?
Because all the answers that the team needed to be successful were not just in the data. The team had to go on-site to empathise and experience the assembly line first-hand to realize where they made a mistake.
No AI or machine could have told the data science team that they needed more than just the right number of staffers. They need to be there on site to see that they also needed to ensure those staffers knew which assembly station to go to and when to get there to ensure a seamless start to the day.
What’s interesting is that once the team had discovered this nuance, the solution was easier to create, faster to implement, and had more significant impact than the machine learning techniques that were used originally to predict the right number of workers necessary to operate the assembly line.
The importance of empathy
This example is the heart of the design thinking methodology and showcases exactly why humans need to be involved at almost every step of problem solving, from ideation to testing.
Machines cannot empathise (at least not yet…), and often it’s necessary to physically experience the situation – use your five senses and really understanding the problem first-hand. With this added insight, the lens to view each problem is sharpened, and the ability to implement transformational results is increased.
With great problem solving comes great thinking and while machines are showing that they are able to think faster, it does not mean that any of that thought is critical or structurally sound.
Human intervention is still a necessity in any problem-solving scenario. The existence and rapid growth of artificial and machine learning technology cannot, and should not, threaten a human’s ability to tackle the world’s greatest challenges and answer the most complex questions using design thinking. It can only enhance it.
Read more: How PlatformE is fighting fast fashion with big data