A deep neural network running on a standard desktop computer is being used to interpret and make sense of highly technical data relating to nuclear events. And in some cases, it is outperforming the best accepted automated procedures and human experts in the field.
Scientists working for the US Department of Energy’s Pacific Northwest National Laboratory (PNNL) are attempting to address the more complicated issues facing both the environment and national security.
These findings are generated by the utilisation of deep learning in order to teach and program machines and systems to learn and make decisions without any external intervention.
As a field of machine learning, deep learning is likely to become the most lucrative AI technology over the next five years, because of the competitive advantage it will provide to those who can master it. Inference engines that adopt such ‘cognitive computing’ techniques are already being widely used by the big internet ecosystems to improve everything from voice interfaces to facial recognition.
Deep learning is likely to become the AI technology that allows cognitive systems to surpass human intelligence for specific applications.
Radioactive emissions are the source of the nuclear events
Signals are pointers to radioactive decays originating from electron emission from excited atoms. When an atom is energetically excited, the electron ascends to a higher energy state. This is subsequently emitted from the atom as radioactive decay in which to electron returns to its original energy state.
How well do you really know your competitors?
Access the most comprehensive Company Profiles on the market, powered by GlobalData. Save hours of research. Gain competitive edge.
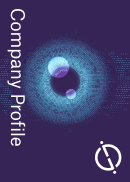
Thank you!
Your download email will arrive shortly
Not ready to buy yet? Download a free sample
We are confident about the unique quality of our Company Profiles. However, we want you to make the most beneficial decision for your business, so we offer a free sample that you can download by submitting the below form
By GlobalDataThe laboratory opened in 2010 and has since captured data from millions of signals originating from radioactive decay events. However, the data is shrouded in external noise, which can hinder the discovery of more uncommon signals. Even a light switch being turned on in a building can produce noise and subsequently affect the data.
PNNL scientist Emily Mace approached some of her colleagues, who are deep learning experts investigating numerous applications leveraging PNNL’s Deep Learning for Scientific Discovery Agile Investment. Mace sent a data package of around two million energy pulse detection events to her colleague Jesse Ward from the underground laboratory.
Mace said: “Some pulse shapes are difficult to interpret. It can be challenging to differentiate between good and bad.”
A neural network is being trained to detect the pulses
Deep learning systems are built using ‘artificial neural networks’ which model the way neurons in the human brain process information. According to Google, “an artificial neural network is trained by showing it millions of training examples and gradually adjusting the network parameters until it gives the classifications we want”.
A properly trained neural network can, therefore, distinguish between signals and general noise. Artificial neural networks, the theory of which has been around for decades, are now possible in practice, thanks to the combination of accelerated computing (i.e. the use of a GPU together with a CPU) with extensive datasets.
A sample of 32,000 pulses was used to adapt the network, programming it to learn the changing features the pulses exhibited that would be critical when interpreting the data. Jesse Ward then sent over thousands of additional pulses so that the network could begin to deduce what signals were good and which were bad; as time progressed, the more complex the pulses became.
The product of this training yielded a network that was able to accurately identify pulse shape events to the same degree as experts, or in some cases, even precede those levels. When the network is fed manageable data, it achieves a 99.9% successful pulse reading.
Ward added: “This is a relatively simple neural network but the results are impressive. You can do productive work on important scientific problems with a fairly primitive machine. It’s exciting to consider what else is possible.”
Related Company Profiles
Google LLC