Without a doubt Artificial Intelligence (AI) has already changed the way consumers interact with technology and the way businesses think about big challenges like digital transformation.
In fact, GlobalData research shows that approximately 50% of IT buyers have already prioritised the adoption of AI technologies. And that number is expected to jump to more than 67% over the next two years.
However, a dark shadow looms over this universal sense of optimism, namely the growing realisation that good AI is hard to come by.
More specifically, the skill and resources required to arrive at a valuable outcome, such as predicting the fastest way to drive home from work, is immense. Worse, such decisions may appear to be correct when in reality they harbour unseen biases (bad assumptions) based on incorrect or incomplete data. And many facets of AI such as Deep Learning (DL) algorithms are in essence a black box, unable to reveal how and why a given decision has been made.
Marketing key to improving trust in AI
Global technology and platform providers with a stake in AI are starting to aggressively address these unseen dangers, shifting their stance away from a “what can AI do for you!” marketing message to a more pragmatic view that priorities the foundations of AI, such as data quality.
Over the last two weeks, two of these vendors, IBM and Google, both took an important, next step by introducing tools capable of building trust and transparency into AI itself. Both offer highly divergent approaches, yet neither solves the problem in its entirety.
How well do you really know your competitors?
Access the most comprehensive Company Profiles on the market, powered by GlobalData. Save hours of research. Gain competitive edge.
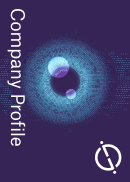
Thank you!
Your download email will arrive shortly
Not ready to buy yet? Download a free sample
We are confident about the unique quality of our Company Profiles. However, we want you to make the most beneficial decision for your business, so we offer a free sample that you can download by submitting the below form
By GlobalDataGoogle’s What-If-Tool
Google’s new tool, appropriately named the What-If Tool, for example, allows users to analyse an machine learning model directly without any programming.
Intended for use long before an AI solution is put into operation, this tool allows users to readily visualise how the outcome of a given machine learning model will change according to any number of “what if” scenarios surrounding the model itself or its underlying data set. The idea is to quickly ferret out programming errors, problems with the data set or even whether or not an algorithm is fair or biased.
But because it is built to be used prior to deployment, it cannot foresee any changes to the underlying data or business parameters that may ultimately impact results.
Conversely, IBM has taken an operational approach to the problem with a new set of trust and transparency capabilities for AI on IBM Cloud.
IBM’s approach
IBM’s new tools evaluate the effectiveness of a given model based on how the business expects it to behave, explaining its effectiveness and accuracy in natural, business language. Moreover, they don’t look at a model at rest but instead evaluate it at run time based on the business KPIs upon which it was founded.
These new features are therefore a living, constant watchdog that evolves with the overall business. However, because these services base their results on anticipated behaviour, they cannot reveal problems such as biases that may be built into the data itself.
There is no one solution
There are several conclusions that can be drawn from these two innovations. First, there is no single, magic wand available to solve this problem in both theory and practice.
Second, technology providers recognise that a lack of trust in the solutions built on their software will ultimately lead to a lack of trust in those vendors.
For that reason, both solutions are being offered free of charge, Google’s as open source and IBM’s as a free add on for existing users. Most importantly, the divergent nature of these solutions point to the necessity of a multi-pronged approach to building trust, first in the underlying data, next in the model and algorithms, and finally in the final solution running in the wild.
Related Company Profiles
Google LLC