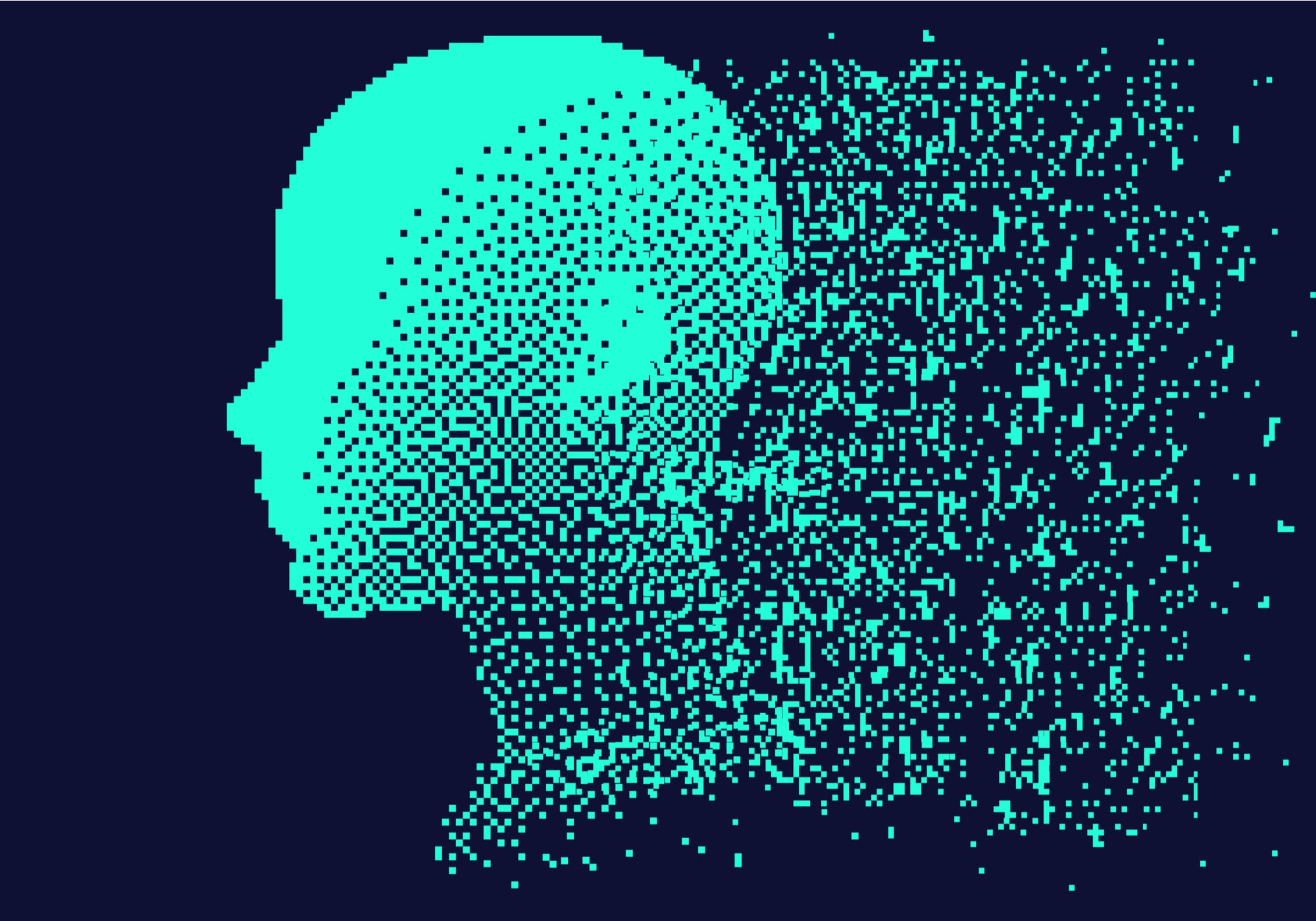
The mind of an experienced and dedicated cybercriminal works like that of an entrepreneur: the relentless pursuit of profit guides every move they make. At each step of the journey towards their objective, the same questions are asked. How can I minimise my time and resources? How can I mitigate against risk? What measures can I take that will return the best results?
Incorporating this ‘enterprise’ model into the cybercriminal framework uncovers why attackers are turning to new technology in an attempt to maximise efficiency, and why a report from Forrester earlier this year revealed that 88% of security leaders now consider the nefarious use of artificial intelligence (AI) in cyber activity to be inevitable. Over half of the responders to that same survey foresee AI cyberattacks manifesting themselves to the public in the next twelve months – or think they are already occurring.
AI has already achieved breakthroughs in fields such as healthcare, facial recognition, voice assistance and many others. In the current cat-and-mouse game of cybersecurity, defenders have started to accept that augmenting their defences with AI is necessary, with over 3,500 organisations using machine learning to protect their digital environments. But we have to be ready for the moment attackers themselves use open-source AI technology available today to supercharge their attacks.
Enhancing the attack life cycle
To a cybercriminal ring, the benefits of leveraging AI in their cyberattacks are at least four-fold:
- It gives them an understanding of context
- It helps to scale up operations
- It makes attribution and detection harder
- It ultimately increases their profitability
To best demonstrate how each of these factors surface themselves, we can break down the life cycle of a typical data exfiltration attempt, telling the story of how AI can augment the attacker during the campaign at every stage of the attack.
Stage 1: Reconnaissance
In seeking to garner trust and make inroads into an organisation, automated chatbots would first interact with employees via social media, leveraging profile pictures of non-existent people created by AI instead of re-using actual human photos. Once the chatbots have gained the trust of the victims at the target organisation, the human attackers can gain valuable intelligence about its employees, while CAPTCHA-breakers are used for automated reconnaissance on the organisation’s public-facing web pages.
How well do you really know your competitors?
Access the most comprehensive Company Profiles on the market, powered by GlobalData. Save hours of research. Gain competitive edge.
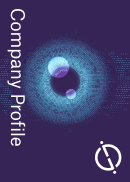
Thank you!
Your download email will arrive shortly
Not ready to buy yet? Download a free sample
We are confident about the unique quality of our Company Profiles. However, we want you to make the most beneficial decision for your business, so we offer a free sample that you can download by submitting the below form
By GlobalDataStage 2: Intrusion
This intelligence would then be used to craft convincing spear-phishing attacks, while an adapted version of SNAP_R can be leveraged to create realistic tweets at scale – targeting several key employees. The tweets either trick the user into downloading malicious documents or contain links to servers which facilitate exploit-kit attacks.
An autonomous vulnerability fuzzing engine based on Shellphish would be constantly crawling the victim’s perimeter – internet-facing servers and websites – and trying to find new vulnerabilities for an initial foothold.
Stage 3: Command and control
A popular hacking framework, Empire, allows attackers to ‘blend in’ with regular business operations, restricting command and control traffic to periods of peak activity. An agent using some form of automated decision-making engine for lateral movement might not even require command and control traffic to move laterally. Eliminating the need for command and control traffic drastically reduces the detection surface of existing malware.
Stage 4: Privilege escalation
At this stage, a password crawler like CeWL could collect target-specific keywords from internal websites and feed those keywords into a pre-trained neural network, essentially creating hundreds of realistic permutations of contextualised passwords at machine-speed. These can be automatically entered in period bursts so as to not alert the security team or trigger resets.
Stage 5: Lateral movement
Moving laterally and harvesting accounts and credentials involves identifying the optimal paths to accomplish the mission and minimise intrusion time. Parts of the attack planning can be accelerated by concepts such as from the CALDERA framework using automated planning AI methods. This would greatly reduce the time required to reach the final destination.
Stage 6: Data exfiltration
It is in this final stage where the role of offensive AI is most apparent. Instead of running a costly post-intrusion analysis operation and sifting through gigabytes of data, the attackers can leverage a neural network that pre-selects only relevant material for exfiltration. This neural network is pre-trained and therefore has a basic understanding of what constitutes valuable material and flags those for immediate exfiltration. The neural network could be based on something like Yahoo’s open-source project for content recognition.
AI cyberattacks: Staying one step ahead
Today’s cyberattacks still require several humans behind the keyboard making guesses about the sorts of methods that will be most effective in their target network – it’s this human element that often allows defenders to neutralise attacks.
Offensive AI will make detecting and responding to cyberattacks far more difficult. Open-source research and projects exist today which can be leveraged to augment every phase of the attack lifecycle. This means that the speed, scale, and contextualisation of attacks will exponentially increase. Traditional security controls are already struggling to detect attacks that have never been seen before in the wild – be it malware without known signatures, new command and control domains, or individualised spear-phishing emails. There is no chance that traditional tools will be able to cope with future attacks as this becomes the norm and easier to realise than ever before.
To stay ahead of this next wave of cyberattacks, AI is becoming a necessary part of the defender’s stack, as no matter how well-trained or how well-staffed, humans alone will no longer be able to keep up. Hundreds of organisations are already using autonomous response to fight back against new strains of ransomware, insider threats, previously unknown techniques, tools and procedures, and many other threats. Cyber AI technology allows human responders to take stock and strategise from behind the front line. A new age in cyber defence is just beginning, and the effect of AI on this battleground is already proving fundamental.
Max Heinemeyer is the director of threat hunting at Darktrace, a cybersecurity firm that leverages AI to defend companies from cyberattacks.Â
Read more: AI cybersecurity: businesses need machines to fight machines