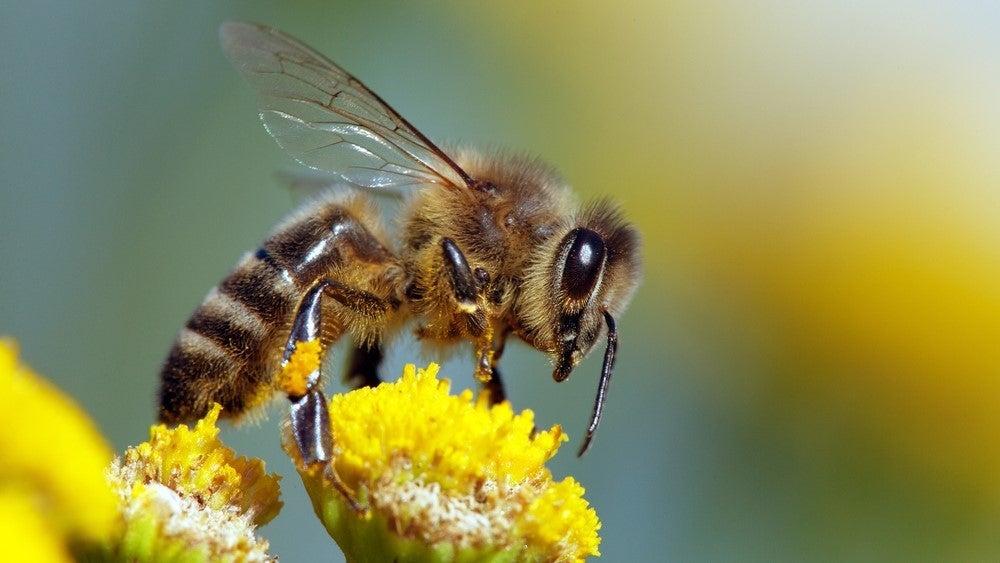
Machine learning models seek to mimic the natural, human ability to process information, enabling technology to take decisions and complete tasks accordingly – and making artificial intelligence (AI) titularly accurate. However, software company Opteran is pursuing a new approach: one of ‘natural intelligence’ modelled on the neural networks of insects.
Artificial Intelligence versus ‘natural intelligence’
Since OpenAI released ChatGPT in 2022, setting the record for the fastest sign up of one million users and supercharging the AI hype, the sector has seen rapid growth and investment. GlobalData predicts that the AI market will be worth $383.3bn by 2030, which would represent a 21% CAGR from 2022.
Explaining the limitations AI faces and the potential solutions offered by a ‘natural intelligence’ model, James Marshall, co-founder and chief science officer at Opteran, tells Verdict: “The difference between a natural intelligence algorithm and an AI algorithm – a deep net – would be that deep nets are usually like a big soup. It’s very loose brain inspiration that is inspired by a very small part of your brain – the visual cortex.
“Having found that that simple model of that part of the brain can do image recognition, the industry has then tried to turn that into a silver bullet that solves all the problems in autonomy, and they’ve had quite a lot of success. However, the challenge is really that most of the success is actually driven by having more data and more compute available. The inputs that are going in are pretty much exponential in growth, whereas the performance improvements are normally linear; that’s why people say AI is running out of steam, because you’re reaching the limits of how big you can make a network or how much data you can push through it.
“Natural intelligence is exactly trying to solve that more holistic problem … It’s moving from having a soup of very simple neurons, which is how you can think about machine learning working, to something much more structured and hence much more efficient with a lot of variety in connection patterns, in the types of neurons and jobs they’re doing. It’s just trying to capture that real variety we see in real brains, which must be there for a reason.
“If you could solve the autonomy problem with just a soupy brain full of all the same neurons just wired together randomly, then that’s how brains would look, but they don’t work that way.”
How well do you really know your competitors?
Access the most comprehensive Company Profiles on the market, powered by GlobalData. Save hours of research. Gain competitive edge.
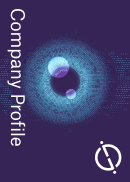
Thank you!
Your download email will arrive shortly
Not ready to buy yet? Download a free sample
We are confident about the unique quality of our Company Profiles. However, we want you to make the most beneficial decision for your business, so we offer a free sample that you can download by submitting the below form
By GlobalDataHoneybee foragers and the ‘third wave’ of AI
AI progressed with the development of machine learning in the 1980s, when the approach moved away from logic programming (the ‘first wave’ of AI), and towards the neural network model that enables computers to learn independently. This ‘second wave’ is described by Marshall as “a kind of cartoon of how the brain works”, analysing data and compute to reach decisions.
Opteran posits that insects could provide an alternative, more efficient – and ultimately more intelligent – model to the “soup of very simple neurons”. Marshall suggests that this approach will shape the ‘third wave,’ translating the structure and function of brains into technology.
“People call it third-wave AI, but we call it natural intelligence, to differentiate it from artificial intelligence, which is mainly deep learning, in most people’s minds,” he says.
A University of Sheffield spin-out, Opteran studied the brains of various insects with a team of computational and wet neuroscientists, behavioural biologists and computer scientists. Marshall explains: “We spent a lot of time looking at insect brains – which are tiny, they’re like a million neurons for a honeybee forager … it can fly about 10km out from the nest in the complex, visual world that we all live in, find something of interest, but then crucially get back to where it came from, and then even communicate where it has been to others – other nest mates – who can then fly out to exactly the same flower patch. They’re amazing visual navigators, and they’re doing that with a brain of a million neurons that occupies a cubic millimetre.
“Now, if you compare that to where our technology currently is, in terms of mapping, localization and autonomy in general, it’s just incomparable. We don’t have any technology that can reach that level of performance. So, the question I started off trying to answer within the university – and what led to the formation of Opteran – was: how can a bee brain do that?
“It’s not just bees, other insects do it – desert ants, for example. They live in the desert where they can’t lay pheromones, which is what people often think about ants doing to navigate around, because the pheromones evaporate as it’s too hot there, so they’re amazing visual navigators as well … We started off trying to unravel this and we use tools like brain atlases that research groups around the world have worked on, neural recordings (which we did ourselves), we even put animals in VR – we put bees in virtual reality, for example. We kind of pieced together quite a lot of the puzzle around how that tiny brain can actually solve this visual navigation problem.”
‘Natural intelligence’ solving problems in practice
Current AI-powered robots still require some human direction to complete a task and are at risk of traffic jams or collisions unless software advancements can enable them to make local decisions. Marshall believes Opteran’s model of ‘natural intelligence’ offers a potential avenue for AI to develop in this way.
He explains: “Brains evolved to solve movement, and everything else that we do that’s more intelligent than movement sits on top of that basic functionality. We’re starting with the same problem; we’ve looked at the lower-level brain functions that govern navigation. That’s good because, as well as being the starting point for autonomy, it’s also a hugely challenging technological area with massive commercial demand.”
The technology has potential in a variety of sectors, but the first commercial uses look likely to be in warehouse robotics, which is proving to be a rapidly growing sector with huge demand. According to GlobalData analytics, sales of industrial robots reached $20.7bn in 2022, making it equivalent to 33% of the $63bn market. By 2030, GlobalData predicts that the industrial robots sector will be worth $45.1bn.
“We’re looking at, for example, right now, warehouse robots, drones …” says Marshall, considering the future of Opteran. “Really, we’re selling the mind, which is a set of algorithms, and that mind can control a drone as easily as it can control a legged robot like a Boston Dynamics dog, or a wheeled robot, like a robot vacuum cleaner, or a driverless car.
“We think of it as a ubiquitous technology, but we’re commercial, so we’ll go after the biggest, easiest commercial prizes early on, and there’s an awful lot around warehouse robotics, for example, because there’s huge unsatisfied demand there. The technology is really expensive to deploy, and not robust enough … The robustness and the efficiency of your system is absolutely essential at the cost of deploying.”